Nature Computational Science: Liu Chao’s group collaborates to reveal multiple mixed motives for human altruistic behaviors and their computational mechanisms
On September 12, 2024, Chao Liu’s group at the State Key Laboratory of Cognitive Neuroscience and Learning and McGovern Institute of Brain Science at Beijing Normal University, in collaboration with Hang Zhang’s group at the School of Psychological and Cognitive Sciences, McGovern Institute of Brain Science, and the Peking University-Tsinghua Joint Center for the Life Sciences at Peking University, published their research results in Nature Computational Science “The ‘motive cocktail’ in altruistic behaviors”. The paper exhaustively explores the multiple socioeconomic motives of people as bystanders in intervening in others’ injustices from the perspectives of social justice and altruism, and how the motives interact with each other to influence individuals’ altruistic behaviors, both in terms of punishing the violators and helping the victims. The study contributes to the understanding of psychological computational mechanisms as well as individual differences in third-party altruistic behaviors, such as bravery, and provides a theoretical foundation for future applications in areas such as altruistic values alignment in artificial intelligence.
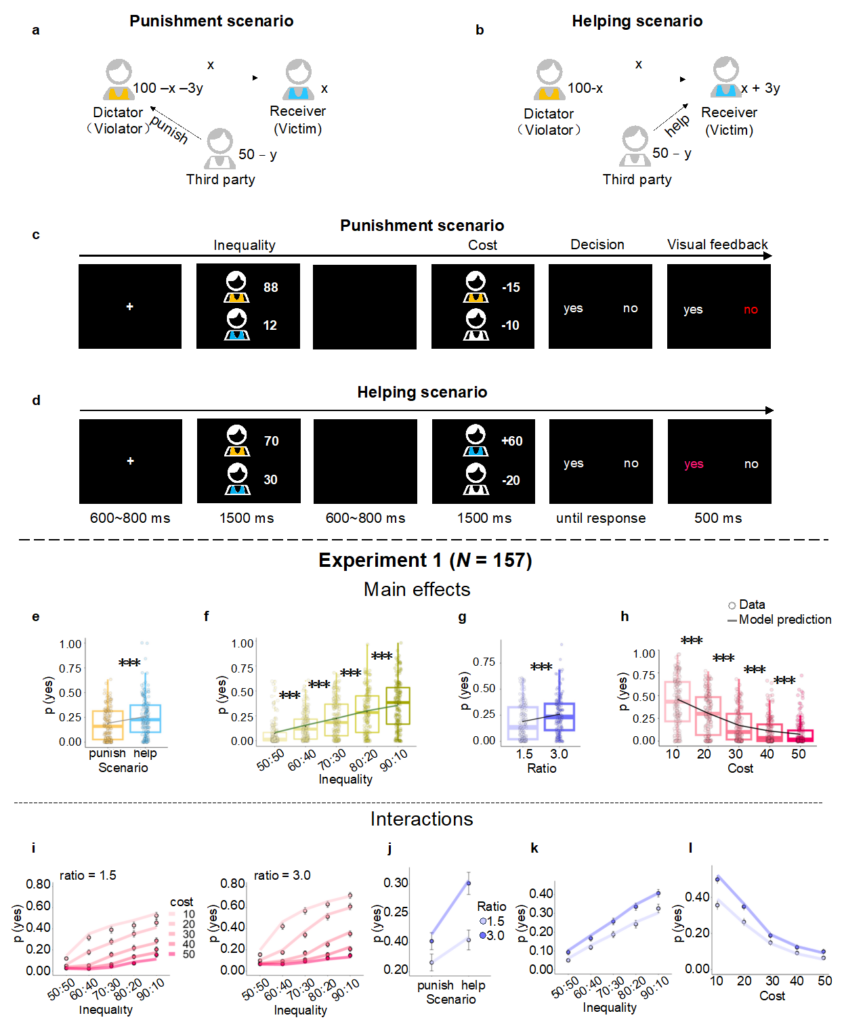
Fairness and justice are important cornerstones for building a harmonious society. When someone in a group violates the principle of fairness and brings harm to others, not only are the victims themselves affected, but a third party as a bystander will often take the initiative to curb the behavior or help the victims, even if the intervention is paid for. Whether the third party punishes the violator or helps the victim, these behaviors are crucial to maintaining and promoting a fair order in society, and thus are also regarded as altruistic behaviors. This study attempts to explore in depth the motivational and psychological mechanisms of third-party altruistic behaviors by incorporating an innovative approach of computational modeling.
Using behavioral experimental data and computational models, the research team reveals how people make decisions when faced with different social situations, taking into account multiple motivational factors (e.g., pursuing fairness, improving efficiency, and maintaining social hierarchies, etc.). The results show that people combine seven different motivations in their altruistic behavioral decision-making process, resulting in multiple mixed motivations, which we call the unifying framework of the “motivational cocktail” model.
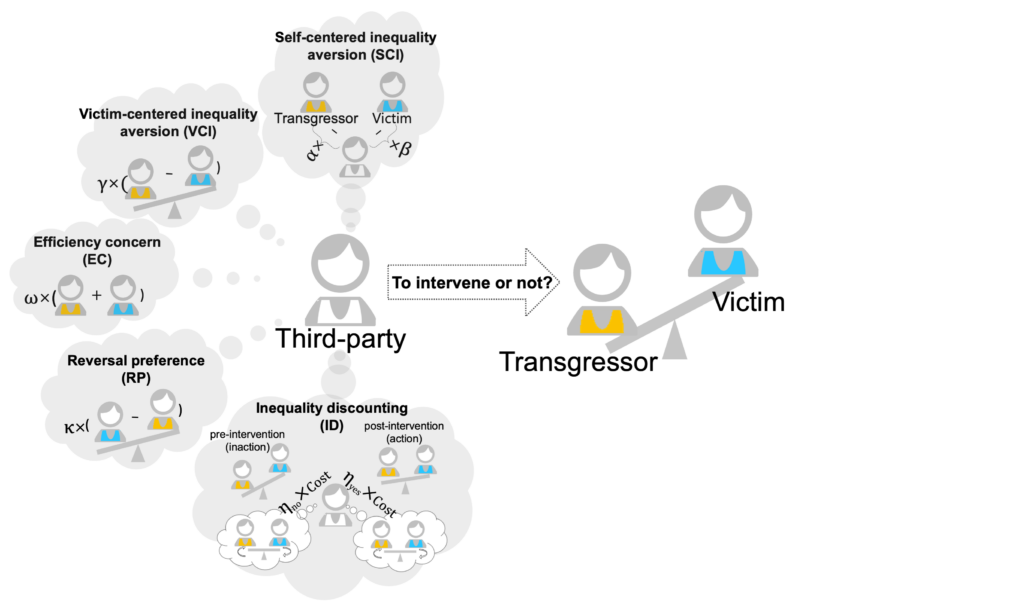
Through cluster analysis, the study identified three different behavioral patterns in the population, namely, justice warriors (35%, eager to pursue fairness), pragmatic helpers (18%, eager to help victims), and rational moralists (47%, more focused on self-interest). Although the altruistic behaviors of all three groups can be optimally explained by the “motivational cocktail” modeling framework, there are differences in the main intrinsic drivers of each group.
The behavior of Justice Warriors is primarily driven by the motivation to ignore remaining injustices among others. They have very high levels of pro-sociality themselves, will show strong reactions to social injustice, and optimistically believe that their actions can eliminate the injustice. The behavior of pragmatic helpers is driven primarily by the motive of inequity-reversal preference; they value the improvement of the victim’s situation and are unconcerned about changing the victim’s relative social rank to that of the offender. Rational moralists’ behavior is driven primarily by the motivation to ignore pre-existing inequities among others. They turn a blind eye to existing inequities among others when the cost of intervention is high. These findings have important implications for understanding the motivational and psychological mechanisms of individual differences in altruistic behavior.
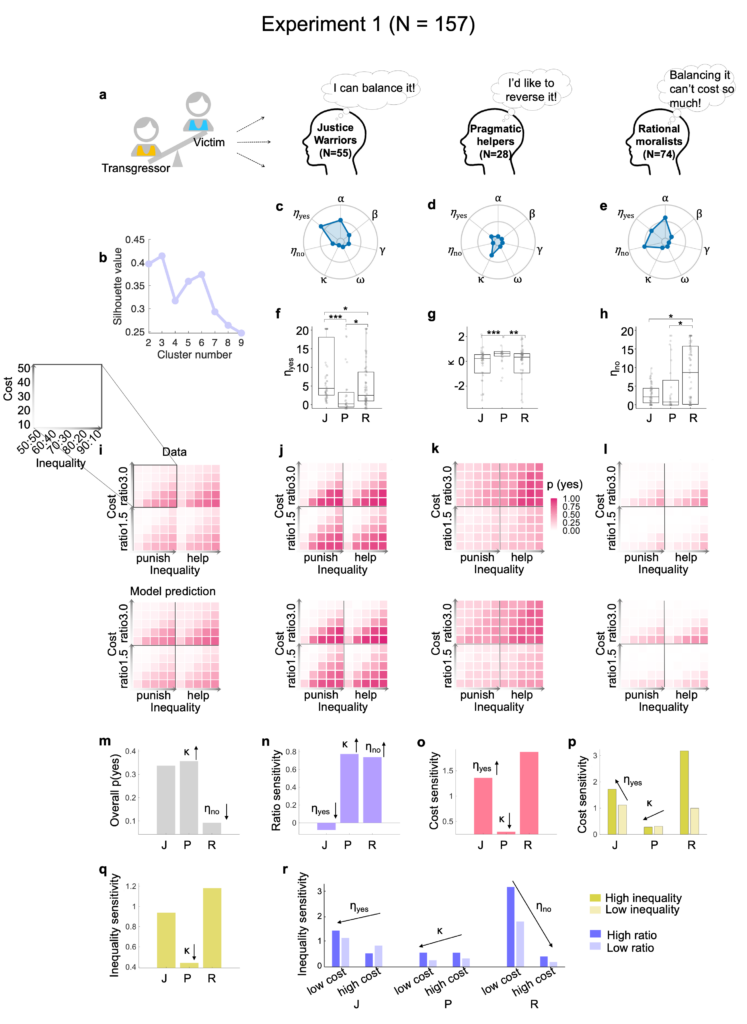
The study also replicated the experimental results through pre-enrollment and online large-sample data collection. The research team collected 1,258 subjects from 66 countries or regions on Prolific’s online platform to repeat the findings, further confirming the reliability and generalizability of the study’s findings.
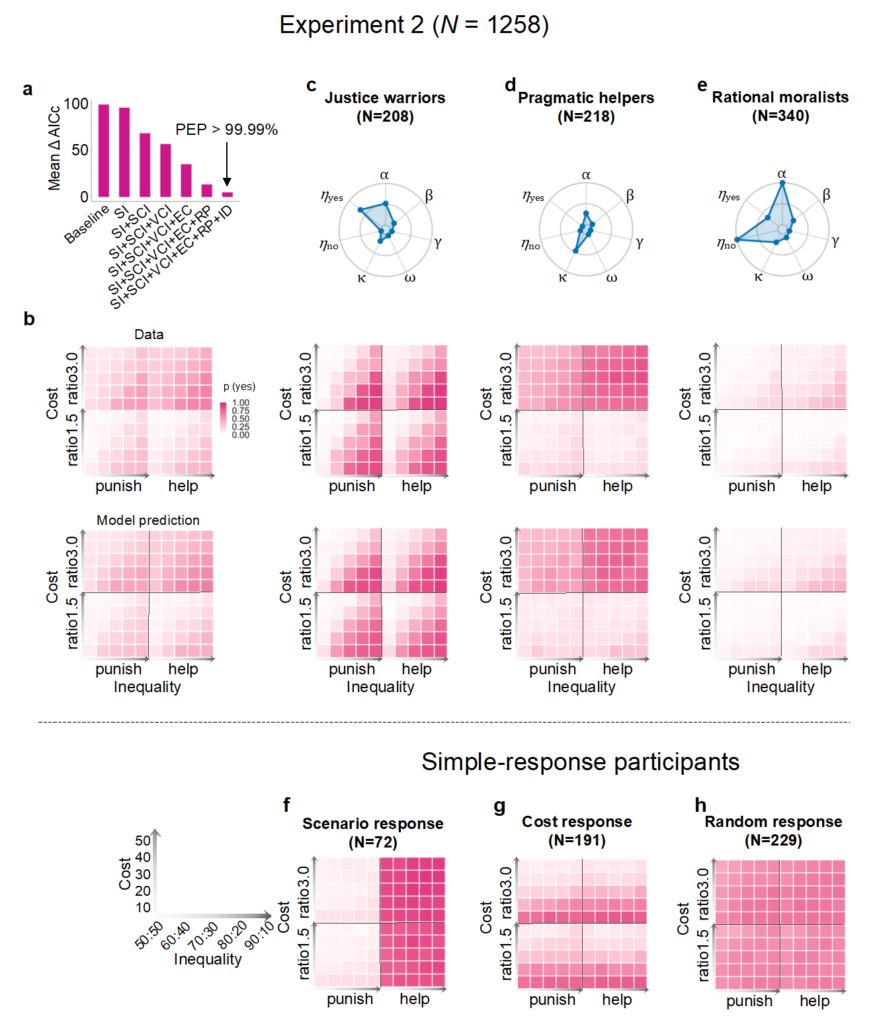
In addition, the “motivational cocktail” integration model successfully reproduced several behavioral findings from previous studies, demonstrating the applicability of the modeling framework in explaining a broader range of altruistic behaviors. For example, the model was able to explain behaviors such as the victim’s direct punishment of the offender (second-party punishment).
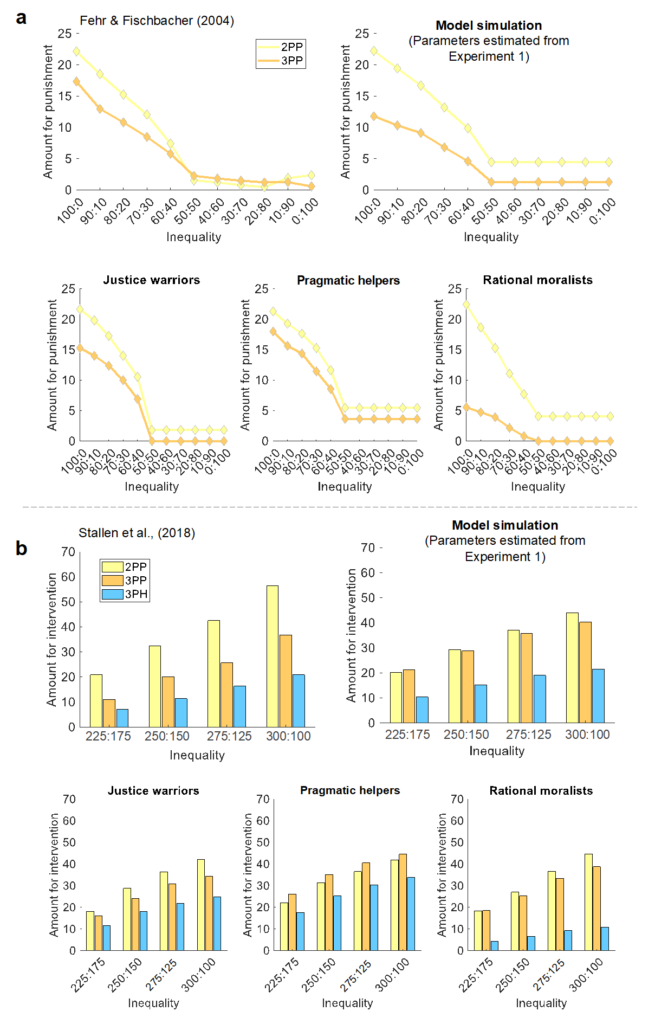
These findings suggest that the framework of the “motivational cocktail” as an integrative model is not only capable of explaining the altruistic behaviors of individuals as third parties, but is also applicable to explaining a wider range of altruistic behaviors as well as individual differences in altruistic behaviors. These findings not only improve our understanding of the complexity of human altruistic behavior, but may also provide a scientific basis for the development of policies that promote social justice and cooperation, and lay the groundwork for future applications in areas such as artificial intelligence and robotics.
The same issue of News & views published a review article “Deconstructing the compounds of altruism” by Hu Jie, a researcher at East China Normal University, which pointed out that the findings of this paper provide a basis for developing more detailed models of human altruistic behavior. By incorporating the complex motivational interactions behind altruistic behavior, AI systems can be trained to make more human-like decisions and act in accordance with human moral values, thus becoming safer and more reliable. This is particularly important for areas such as AI safety, value alignment, autonomous systems, robotics, and AI-powered decision-making tools.
Xiaoyan Wu, a PhD student in Chao Liu’s group, and Xiangjuan Ren, a graduated PhD student in Hang Zhang’s group at Peking University, co-authored this paper, and Chao Liu and Hang Zhang, a researcher at Peking University, are the co-corresponding authors. This study was supported by the Science and Technology Innovation 2030 – “Brain Science and Brain-like Research” Major Project (2021ZD020050), the National Natural Science Foundation of China (32171095, 32271092, 32130045), the Beijing-Tsinghua Life Science Research Center, the National Social Science Foundation of China (19ZDA301), and the National Academy of Sciences of China (19ZDA301), as well as the National Science Foundation of China (19ZDA301). Foundation Major Project (19ZDA363) and other programs.
Link to original article:
Wu X, Ren X, Liu C* , Zhang H* (2024). The “motive cocktail” in altruistic behaviors. Nature Computational Science. https://www.nature.com/articles/s43588-024-00685-6
Link to same comment:
Hu J (2024). Deconstructing the compounds of altruism. nature Computational Science. Nature Computational Science. https://www.nature.com/articles/s43588-024-00690-9
Subject Link:
Liu Chao Subject Group: https://liuchaolab.bnu.edu.cn/
Previous Related Papers:
1) Wang H, Wu X, Xu J, Zhu R, Zhang S, Xu Z, et al. (2024). Acute stress during witnessing injustice shifts third-party interventions from punishing the perpetrator to helping the victim. PLoS Biology 22(4). e3002195. https://doi.org/ 10.1371/journal.pbio.3002195
2) Jin K, Wu J, Zhang R, Zhang S, Wu X, Wu T, Gu R, Liu C. (2024). Observing heroic behavior and its influencing factors in immersive virtual environments. Proc Natl Acad Sci U S A. 2024 Apr 23;121(17):e2314590121.
3) Wang, H., Zhen, Z., Zhu, R., Yu, B., Qin, S., & Liu, C. (2022). Help or punishment: acute stress moderates basal testosterone’s association with prosocial behavior. Stress, 25(1), 179- 188.
4) Zhen, Z, Wang, H., Zhu, R., Zhang, S, Jin T, Qin, S., Liu, C. (2021). Acute psychosocial stress increases third-party helping but not punishing behavior. Stress 24 (4), 430-441.